Article • Imaging in characterisation and classification of tumour types
Taking a closer look at breast cancer
Breast cancer has no “one size fits all” therapy approach: subtypes differ significantly in malignancy, progression, and treatment response. Therefore, the more is known about the type of carcinoma in a patient, the better the outcome. At the annual scientific EUSOBI meeting in Valencia, Dr Ramona Woitek pointed out the potential of novel imaging techniques and computational image analysis for classification and characterization of breast cancer as well as treatment response prediction.
Report: Wolfgang Behrends
Image source: Leithner et al., Breast Cancer Research 2019 (CC BY 4.0)
Molecular imaging can provide valuable insights into cancer characteristics, Dr Woitek, a Professor of Radiology at the Research Center for Medical Image Analysis and AI (MIAAI) at Danube Private University in Krems, Austria, outlined. It can help differentiate cancers via hallmarks such as increased self-proliferation, deregulated cellular metabolism and tumour vasculature. In a best-case scenario, imaging can help identify – potentially actionable – mutations, and select those patients who will benefit the most from undergoing tumour tissue sequencing.1
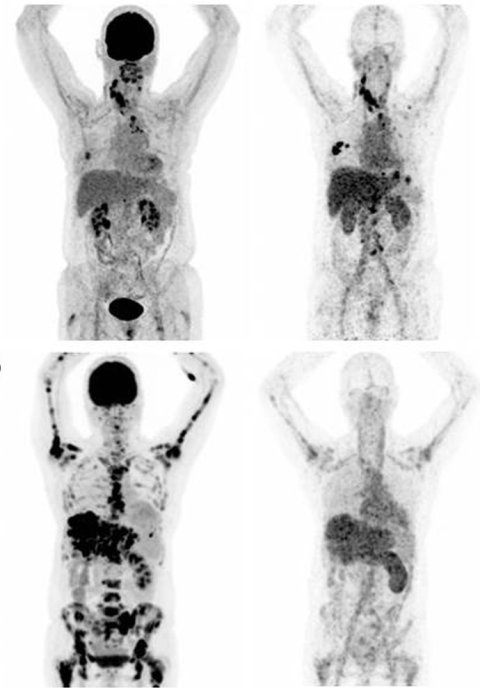
Image source: Bensch et al., EJNMMI 2018 (CC BY 4.0)
Beyond standard-of-care imaging such as conventional mammography, ultrasound and MRI, functional imaging can provide more specific information, the expert explained: 18F-FDG PET is mainly used in metastatic breast cancer, but can also help understand a tumour’s microenvironment and biology, while 18F-FLT PET and 18F-FMISO PET show cell proliferation and hypoxia in tumours, respectively.2 Another tool that can help make better treatment decisions is 89Zr-Trastuzumab, a tracer that identifies tumour heterogeneity between metastatic lesions.3 Waiting in the wings are advanced MRI techniques using hyperpolarised carbon-13 (13C) and sodium (23Na), which show promise in treatment response prediction and early response detection by distinguishing between actual progression and pseudoprogression.4,5
Semantic descriptors in MRI images serve as an effective way to distinguish between breast cancer subtypes, Woitek pointed out. ‘It has been shown in several studies that some of these descriptors are more frequently found in certain subtypes.’6,7 These distinctions can provide valuable and actionable information, especially in settings where assessment of the KI-67 marker is not available. ‘For example, irregular borders and persistent enhancement are most frequently found in Luminal A cancer types, while Luminal B is characterised by more heterogeneous enhancement. HR-/HER2+ breast cancers typically feature smooth borders, washout, and are more frequently associated with multifocality.’
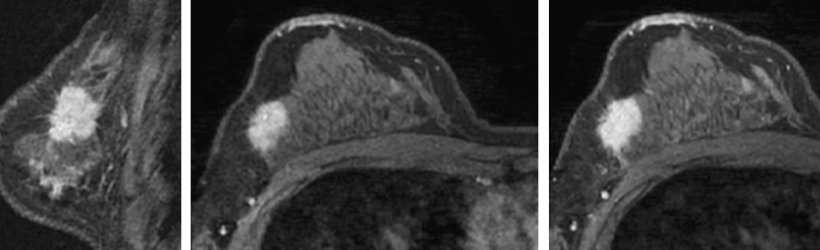
Image source: Kawashima et al., SpringerPlus 2014 (CC BY 4.0)
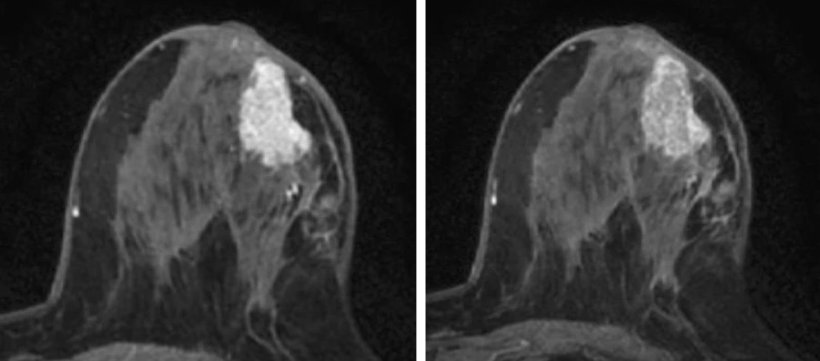
Image source: Kawashima et al., SpringerPlus 2014 (CC BY 4.0)
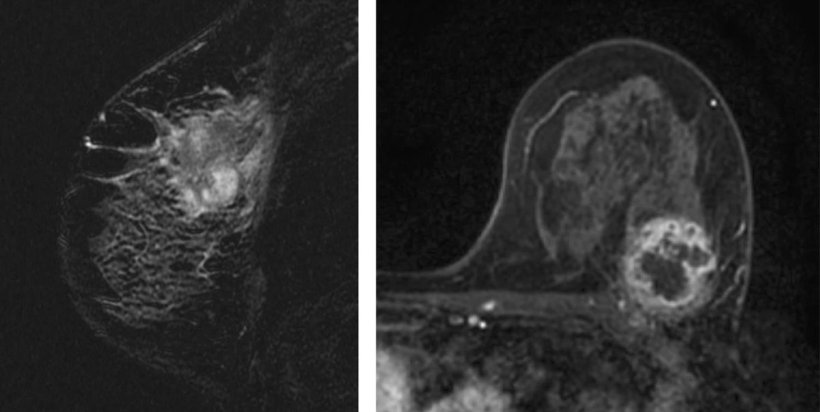
Image source: Kawashima et al., SpringerPlus 2014 (CC BY 4.0)
Heatmaps shed light into the algorithm’s black box
These insights can be further exploited via radiomics, where quantitative features such as shape, volume or texture of a tumour can be extracted. Using dynamic contrast-enhanced MR imaging (DCE-MRI), this provides high-accuracy information especially for distinguishing luminal B-type cancer from other forms,8 often outperforming semantic or clinical features, Woitek reported.
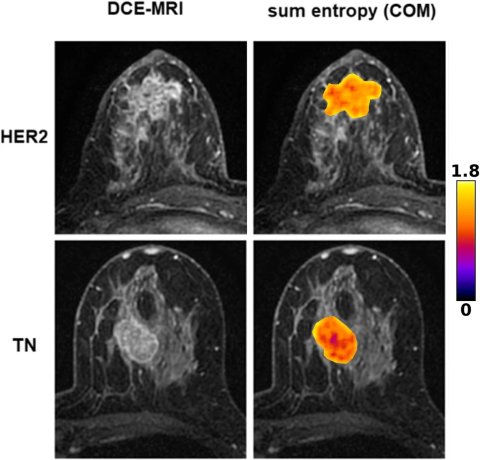
Image source: Leithner et al., Breast Cancer Research 2019 (CC BY 4.0)
Using convolutional neural networks (CNN), high-quality information can also be extracted from multimodal ultrasound imaging (B-mode, colour Doppler and shearwave elastography).9 ‘These networks use deep learning to find the relevant features to classify the tumours by themselves, and they are getting better and better at this. Usually, the more types of data from different sources and the more modalities are put together, the higher the chances of developing really good predictors.’ As with most AI algorithms, this approach is somewhat of a black box. However, this can be overcome using special heatmaps. These class activation maps show how much any pixel of a given diagnostic image contributes to the algorithms classification or prediction, leading to interpretable results. For example, if the algorithm derives its findings mostly from pixels which are far away from the actual tumour site, it is likely that the interpretation is not trustworthy. On the other hand, if the heatmap stays focused on the cancer, the result has high predictive value.
A more profound understanding of cancer types
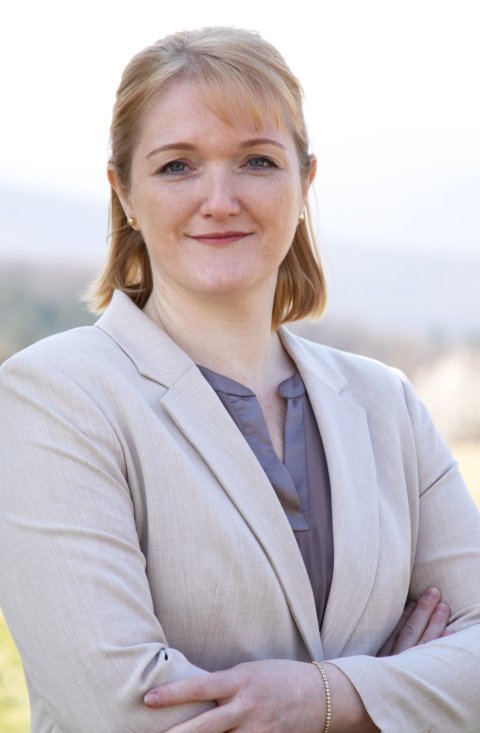
Image souce: Danube Private University
‘Usually, oncologists’ decisions on whether or not to give targeted anti-HER2 treatment are based on single biopsies from single metastatic sites or even from the primary tumour,’ Woitek pointed out. ‘But scans and tracers like this really allow us to get an idea about the heterogeneity of cancer, the receptor status and the expression of HER2 in different sites of the body, and that can actually have a huge effect on treatment decisions.’
Computational image analysis, combined with novel imaging techniques, holds great potential to help characterize cancers, the expert summarized. ‘Especially with novel treatments, our focus on functional techniques will grow. It is our task to identify the key questions we want to answer in the prediction, classification and characterization of cancer, and how this can have the biggest impact on our patients and improve their outcomes.’
Literature:
- Duffy et al.: Mutant p53 in breast cancer: potential as a therapeutic target and biomarker; Breast Cancer Research and Treatment 2018
- Carmona-Bozo et al.: Hypoxia and perfusion in breast cancer: simultaneous assessment using PET/MR imaging; European Radiology 2021
- Bensch et al.: 89Zr-trastuzumab PET supports clinical decision making in breast cancer patients, when HER2 status cannot be determined by standard work up; EJNMMI 2018
- Gallagher et al.: Imaging breast cancer using hyperpolarized carbon-13 MRI; PNAS 2020
- Zaric et al.: Quantitative Sodium MR Imaging at 7 T: Initial Results and Comparison with Diffusion-weighted Imaging in Patients with Breast Tumors; Radiology 2016
- Kawashima et al.: Magnetic resonance imaging features of breast cancer according to intrinsic subtypes: correlations with neoadjuvant chemotherapy effects; SpringerPlus 2014
- Grimm et al.: Relationships Between MRI Breast Imaging-Reporting and Data System (BI-RADS) Lexicon Descriptors and Breast Cancer Molecular Subtypes: Internal Enhancement is Associated with Luminal B Subtype; The Breast Journal 2017
- Leithner et al.: Radiomic signatures with contrast-enhanced magnetic resonance imaging for the assessment of breast cancer receptor status and molecular subtypes: initial results; Breast Cancer Research 2019
- Zhou et al.: Decoding the molecular subtypes of breast cancer seen on multimodal ultrasound images using an assembled convolutional neural network model: A prospective and multicentre study; EBioMedicine 2021
28.02.2024