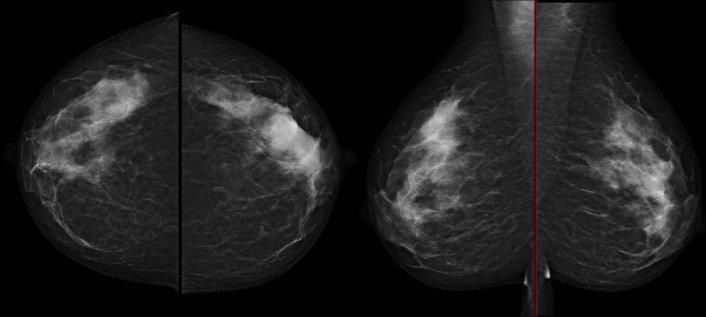
Article • Algorithms must meet quality criteria
Deep Learning in breast cancer detection
A French expert in breast imaging looked at the latest Deep Learning (DL) applications in her field, screening their strengths and weaknesses in improving breast cancer detection.
Report: Mélisande Rouger
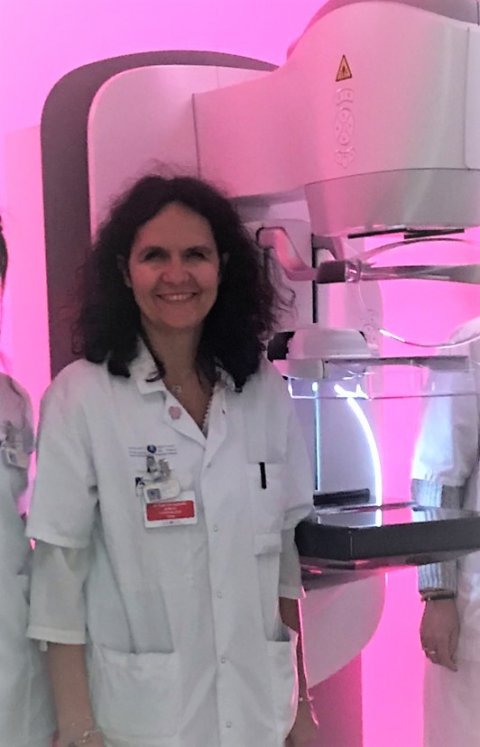
It is really important to understand which types of data sets need to be checked when evaluating an AI model for image interpretation, according to Isabelle Thomassin-Naggara, Professor of Radiology at Sorbonne University in Paris. ‘The model is usually built on enriched data cohort with a high volume of BCs, ideally including all types of BC on mammography, speculated mass, run mass and cluster classification. Another data set must be used for internal validation,’ she said.
The model also needs to be externally validated in another cohort with lower prevalence of cancer and possibly with more subtler cancers to improve its accuracy. Last but not least, the model must be evaluated with an independent data set with a prevalence that is representative of the screened population. ‘Today, an algorithm needs more than one million mammographiesto truly demonstrate its efficiency. The development of dedicated platforms must be elaborated to build larger evaluation of data sets that are more representative of the population. All these steps can be performed retrospectively, but, before clinically validating a model, a randomised trial comparing the accuracy of a model with the radiologists’ is needed,’ she said. Incremental improvement in the ‘area under curve’ (AUC) is not directly translatable to improve patient outcomes in a clinical setting and it is uncertain which examined population a commercially AI system would flag as having more than 2% of malignancy requiring additional diagnostic workup in current clinical practice.
A costly mistake
‘We learned from our experience of CAD in mammography that a product that promises new technologies too quickly can be a costly mistake later, by leading to find more false positives without improving cancer detection,’ she said.
A number of studies have compared human vs. AI performance. A recent publication in the Lancet compared 25 studies and highlighted methodological limitations of validation studies of AI models, including the absence of calculation of number of subjects, absence of prospective design, presence of many statistic biases, absence of validation on independent samples and absence of comparison on the same population of AI model and human reading.
Using the AI models and human reading showed better outcome than using AI or the radiologist alone
Isabelle Thomassin-Naggara
The Google paper on how its DL algorithm beat radiologists at detecting cancer made the news early this year and frightened a lot of radiologists. But this publication showed a number of significant limitations. ‘When you look at this publicity, you can pick major errors. Look at the quality of the mammogram: even an expert radiologist would not be able to make a diagnosis correctly from such a low quality image,’ said Thomassin-Naggara. She also pointed out the methodology, which used two data sets with very different prevalence of malignancy – 1.6 % in the training cohort vs. 22.2% in the validating cohort. The vast majority of images in this study were acquired on Hologic equipment, so did not represent manufacturers’ diversity. Last, out of the +25,000 women included in the cohort, only 500 cases were used to compare the AI model with the human readers.
However, the main limitation was probably the poor performance of human readers overall, rather than a clearly spectacular performance from AI. ‘Human readers’ performance was very low and this could be improved,’ she said. By contrast, another publication used two data sets with a normal prevalence of BC in screening populations in the US and independent validation cohorts in Sweden. ‘Four AI models were tested and the results are interesting: No single AI model out-performed the radiologists. Using the AI models and human reading showed better outcome than using AI or the radiologist alone,’ she said. These results are in line with previous work on an enriched cohort, which showed that radiologists’ performance with AI was better than without. ‘We have an increased specificity and sensitivity when the radiologist is helped by AI, and no limitation of time duration, which used to be a restriction with CAD,’ she added.
Improving image acquisition and patient pathway
The challenge in these two applications is to lower radiation dose. Some software can do scatter correction without using anti-scatter grid, by identifying the structures that cause scatter and subtracts the calculated scatter. The dose-saving depends on breast thickness and structure. Another AI model has been developed to help the technician to achieve optimal positioning, by defining the right compression force and indicating the right exposure parameters. After each exposure, anonymised data are sent to an external cloud database, which can analyse the final image quality of each diagnostic image and give advice on all acquisition parameters.
Optimising the patient pathway with AI-enhanced imaging delivers the ability to automatically evaluate breast density and quickly determine which patients will need ultrasound after mammography. Up to now, the different solutions to automatically assess breast density displayed low reproducibility and low performance, especially new BI-Rads lexicon because these softwares were based on segmentation.
Recently, two DL softwares were optimised and demonstrated very nice agreement, reaching 99% for MLO and 96% for CC view to distinguish between fatty and dense breast. Another publication showed very good kappa agreement with radiologists (0.85)
The French radiology community reently highlighted the need to develop cloud software to automatically analyse daily images that can detect defaults or instabilities, to help medical physicists: decrease the analysis time during quality control (QC); create automatic analysis of QC criteria to simplify daily practice; offer automatic patient quality control assessment for technologists, for example blurring, position, compression, artefacts; and create a report for technologists’ self-assessment. ‘AI with DL will change practice and the place of imaging on the patient pathway. Imaging-based risk prediction of BC and biomarkers are potentially fantastic ways to personalise medicine and improve recommendations to our patients,’ Thomassin-Naggara concluded.
Profile:
Isabelle Thomassin-Naggara is Professor of Radiology MD at APHP Sorbonne University in Paris, France
23.10.2020